Combining machine learning, ecological modelling and plant morphological characteristics to develop a tool for identifying plants of Switzerland
Philipp Brun
Patrice Descombes
Lucienne Claudine de Witte
2020 - 2022
Cooperation FinancingCombining machine learning, ecological modelling and plant morphological characteristics to develop a tool for identifying plants of Switzerland
Aim of the project
Recent advances in image classification have fostered the advent of user-friendly mobile apps helping amateurs to identify species from images with great potential for citizen science. These apps are working well for common species with distinct morphological features. However, they tend to perform badly for less common species and for groups of species with very similar morphological characteristics. Many Swiss plant species, for example, are not recognized well by the current apps. Here, we propose a novel approach combining machine learning-based image recognition with spatially explicit ecological and morphological meta-information for the identification of the c. 4'000 Swiss plant species from georeferenced pictures. This combination is expected to considerably improve species identification as new images are not only classified according to visual features but also with regards to ecological and geographical plausibility. This will greatly aid data acquisition by citizen scientists.

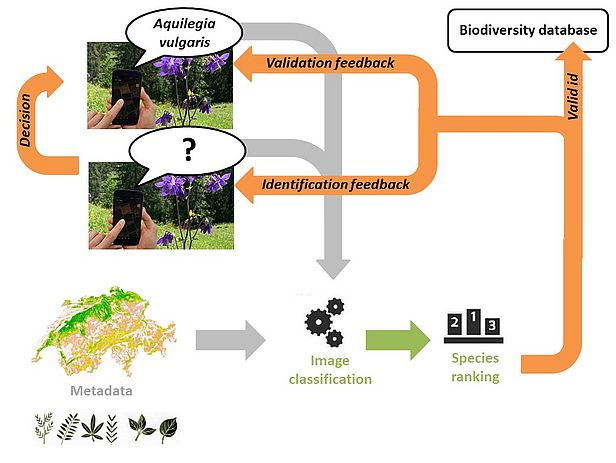
May 2022
We have now trained a fifth generation of the COMECO classifier with 1.19 million images and 8.04 million occurrence observations of 2’516 species. In the image database there are 35'746 new images donated by the project team of „Flora des Kantons Zürich“, 5'917 new images submitted to Info Flora via FlorApp and 184’882 additional GBIF images available since December 2021. In this generation we started validating the classifier with manually-selected test images. So far this was possible for about one third of the species. In addition, we considered the entire photo for evaluation, not just on the center crops.
Now, the classifier correctly identifies 77.7% of single test images, while for 93.2 % of single test images the correct species are within the top five suggestions by the model. For observations with several images (typically three), the identification accuracy increased to 84.4% (top 1) and to 96.8% (top 5). The inclusion of locality information increased the identification accuracy by a similar margin as in previous generations to 81.8% top-1 accuracy and 95.0% top-5 accuracy, for single test images, and to 85.4% top-1 accuracy and 97.2% top-5 accuracy for observations with several images. An updated overview over the classification accuracies on the species-level is provided in this PDF document.
The clear improvement in accuracy when partly considering manually-selected test images of decent quality indicates that previous assessments based on randomly-selected test images underestimated the true skill of the classifier. The main reason for this underestimation is that randomly-selected test images also include bad-quality photos, e.g., due to blurring, or images with the wrong content, such as misidentifications. We will therefore continue to manually select test images in the coming months. Moreover, we will continue with our field campaign to complement the image database with photos of those species that are still underrepresented.
Januar 2022
During the New Year break we have trained a fourth generation of the COMECO classifier with 1.17 Mio images of 2’533 species. In the image database we now also include 9’200 images of 265 hard-to-identify species made by the COMECO team, 57’471 images submitted to Info Flora via FlorApp and 158’226 additional GBIF images available since August 2021.
These additional images resulted in numerous new species included that are rather difficult to identify, such as grasses from the genus Festuca. We therefore expected a somewhat lower overall accuracy of the classifier as compared to the last generation. Anyhow, the classifier has reached a similar identification accuracy as before and correctly identifies 73.0% of test images, while for 90.9% of the test images the correct species is found within the five top hits. From these results we conclude that the many additional images considerably improved the identification accuracy.
The inclusion of locality information increased the identification accuracy by a similar margin as in previous generations to 77.3% and 92.8%, respectively. An updated overview over the classification accuracies on the species-level is provided in this document.
In the coming months, we primarily work on the detailed manual selection of test images. With this selection, we will also be able to test what type of images (e.g. vegetative parts, flowers, fruits) yield most accurate identifications.
During the field season 2021, the COMECO team has captured thousands of images for roughly 300 species on its priority list. This encourages us for the upcoming field season to cover many of the remaining priority species. Still, we rely on expert contributions for the more difficult genera. Therefore, we would like to request botanists to submit more images from their own databases. High priority taxa for example are species from Poales, Apiaceae and Asteraceae as well as from the genera Alchemilla, Campanula, Euphorbia, Euphrasia, Gentiana, Ranunculus, Salix and Saxifraga.
Further information on priority species and on what to photograph can be found in section «Supporting the project». Please don’t hesitate to contact us with your questions or images via email: luciennec.dewitte_at_wsl.ch
To satisfythe growing public interest in the COMECO project and to inform citizen scientists on how they can benefit and contribute, we offer public presentations on the project and public excursions where we explain the tools needed for submitting good plant observations and give advice on how to take good plant images. There will be a free webinar by Info Flora on how to use «FlorApp» for field observations and uploading images on March 11th, and in the afternoon of April 1st we will go on excursion with the Botanical Society in Basel.
End of June 2021
We have just finished the calculations for the third generation of neural nets. This month, the following developments were made:
- Inclusion of all InfoFlora observations and images that were made between April 23rd and May 26th
- Inclusion of manual crops for images of all species (max. 20 per species)
- Inclusion of 1.5 million images that are stored in publicly accessible biodiversity databases. This way we could substantially improve the data basis in particular for wide-spread and cultivated species.
- Improvement of the neural net for image preselection: for each image we conduct a preliminary analysis in order to identify its quality and suitability, and to filter out for example blurred images or images of landscapes. This improved preselection also is somewhat stricter than the former one, which means that now a smaller part of the images is used in the main analysis.
With these extensions we could considerably increase the size of our image database and improve its quality. We now have almost one million suitable training images of 2350 species, 651 species more than in the previous month. The new image classifier correctly identifies 72.9% of test images, and for 90.4% of test images the correct species is among the five species considered most probable by the classifier. When locality information is also considered, these statistics increase to 77.7% and 93.0%, respectively. An updated overview over the classification accuracies on the species-level is provided in this document. Further information on the statistics used and priority species for photographing can be found under the 'Priority'-Tab in the section 'Supporting the project'.
Beside some minor improvements our next steps include a detailed, manual selection of test images, in order to be able to obtain a good understanding in what cases identifications are more or less accurate. Moreover, we will test in for which species images in public biodiversity databases are particularly suitable, and where there may be problems, and we will collect as many good images as possible of species for which the identification is not yet accurate.
Zehnter Mai 2021
This weekend we have finalized the second generation of neural networks. The developments compared to the previous month are:
- Inclusion of all InfoFlora observations and images made between March 9 and April 23.
- Inclusion of images of the atlas of the flora of the Canton Vaud as training images.
- Inclusion of manual crops of InfoFlora images for about 1000 species.
This increase of the database and the improvement of its quiality led to an increase in the accuracy of our neural nets. The updated image classifier now correctly identifies the species for 74.4% of test images, and for 91.1% it suggests the correct species among the top 5 species considered most likely. When combining image and locality infomration, we achieve an accuracy of 78.3% and 93.1% for the corresponding statistics. If the image classifier is provided with two images to classify an observation, it corretly identifies the species in 85.3% of cases. Moreover, in this iteration we could increase the number of species, for which we have sufficient image material, by 40 to 1699. The newly added species include:
- Anemone blanda Schott & Kotschy
- Anthriscus caucalis M. Bieb.
- Armoracia rusticana G. Gaertn. & al.
- Asparagus officinalis L.
- Asplenium billotii F. W. Schultz
- Aubrieta deltoidea (L.) DC.
- Butomus umbellatus L.
- Camelina microcarpa DC.
- Cistus salviifolius L.
- Clypeola jonthlaspi L.
- Cotoneaster salicifolius Franch.'
- Diplotaxis muralis (L.) DC.
- Dorycnium herbaceum Vill.
- Draba tomentosa Clairv.
- Drosera ×obovata Mert. & W. D. J. Koch
- Euphorbia myrsinites L.
- Forsythia ×intermedia Zabel
- Galega officinalis L.
- Gentiana insubrica Kunz
- Hemerocallis fulva (L.) L.
- Himantoglossum robertianum (Loisel.) P. Delforge
- Hymenolobus pauciflorus (W. D. J. Koch) Schinz & Thell.
- Isopyrum thalictroides L.
- Lathyrus sphaericus Retz.
- Laurus nobilis L.
- Lonicera nitida E. H. Wilson
- Lythrum portula (L.) D. A. Webb
- Muscari armeniacum Baker
- Myosotis discolor Pers.
- Nigella damascena L.
- Peucedanum venetum (Spreng.) W. D. J. Koch
- Pisum sativum L.
- Potentilla heptaphylla L.
- Primula acaulis × veris
- Pteris cretica L.
- Quercus cerris L.
- Scilla siberica Haw.
- Sisymbrium irio L.
- Spirodela polyrhiza (L.) Schleid.
- Symphytum bulbosum K. F. Schimp.
- Thuja plicata D. Don
- Veronica praecox All.
- Viola collina Besser
(Three species fell below the limit of 30 observations with images, after modifications on the database.) A detailed overview over classification accuracies on the species level is provided in this document. Further information on the statistics used and priority species for photographing can be found under the 'Priority'-Tab in the section 'Supporting the project'.
It is important to note that these are preliminary results that have to be taken with a grain of salt. In the coming months we are going to clean and adapt training and in partiuclar test data thoroughly and therefore the quality scores may be changing distinctly, in particular on the species level. The information on whether or not sufficient suitable images are available for the different species is a bit more reliable.
Easter 2021
We have just trained the first set of neural nets and already achieve a decent classification accuracy for 1659 species. Based on image information only, the best net so far identifies 72.2% of test images correctly, and for 90.4% of test images the correct species is among the five species, that are estimated as the most probable ones by the net. When including locality information as well, the statistics increase to 76.0% for correct classifications, and 92.4% matches within the five most probable suggestions. Even though these numbers are encouraging, they also show that for many species classifications are not yet functioning well. Moreover, the image material is currently insufficient for any assessment in the case of almost 2000 species. A detailed overview over classification accuracies on the species level is provided in this document. Further information on the statistics used and priority species for photographing can be found under the 'Priority'-Tab in the section 'Supporting the project'.
It is important to note that these are preliminary results that have to be taken with a grain of salt. In the coming months we are going to clean and adapt training and in partiuclar test data thoroughly and therefore the quality scores may be changing distinctly, in particular on the species level. The information on whether or not sufficient suitable images are available for the different species is a bit more reliable.
General
Why we need support from Citizen Scientists
In order to develop a reliable algorithm, we need about 100 high-quality images for each Swiss plant taxon, no matter whether it is native, naturalized, or cultivated. To this end, we use the anonymized observations of the Info Flora database, which currently contains about 500'000 plant images. For a few, widespread species we already have lots of images, yet for the vast majority of species the numbers are far lower than 100 (see the tab «Priority taxa»). The success of this project will therefore depend to a large part on how many high-quality plant images we receive from botanists Citizen Scientists troughout the season 2022.
How you can support us
This project is run in collaboration with Info Flora who provide database and infrastrucutre. Info Flora offers a user-friendly platform for Citizen Scientists to make observations of plant species in the field, including image evidence, in a standardized way. To this end, in particular the «FlorApp» for smart phones is used, which allows making observations directly in the field (see online manual). Those who prefer not to use smartphone cameras to take pictures of plants can complement the observations taken in the field convienently at home, using the «Online-Fieldbook».
If you own a large and informative image data base, e.g. with over 1000 images, that you would like to share with us, please contact us directly via email: luciennec.dewitte_at_wsl.ch. This only applies to images that are not uploaded to the Info Flora data base and that are labelled with species names.
How the community benefits from your help
The classifier we develop in this project will become part of an identification module of the «FlorApp» and thus it will be freely avaiable to everyone. This identification module will provide real-time support to those interested in the identification of plant speices in the field and an opportunity for Citizen botanists to efficiently improve their skills. The more images we receive, the better will be the guidance of the algorithm.
Priority taxa
Priority for photographing are species for which we currently have fewer than 30 observations with suitable images or which currently can only be identified inaccurately by the classifier. Here is a PDF file listing all species meeting these criteria, whereby we used a Top1 accuracy of the image-only classifier of less than 50% as quality criterion. Please note that the quality-scores are preliminary and will likely change in later versions of the classifier. In the coming months, we are going to further refine the priority critera, constraining priority taxa to a few hunderd key species. If you prefer to work with the quality data in a tabular form in order to be more flexible with data manipulation, you may use this XLS file of the full quality list.
Explanations to the documents
In order to assess classification accuracies, we sort out at least five images for each species, so that the algorithm does not 'see' them during the training phase. For each of these photos the algorithm then calculates in the test phase, with which probability the different species are depicted. From this, we deduce the following statistics:
Top 1 : The species, for which the algorithm assigns the highest probability, is the correct one.
Top 5 : The correct species is among the five species, for which the algorithm assigns highest probabilities.
Ungenügend Bildmaterial / Too few images (darkred circles) : There are fewer than 30 observations with suitable images available for the species. Image suitability is assessed prior to classification in an automated suitability assessment. The criteria used for this assessment are described in the tab 'How to take good pictures'. The diameter of the rings represents the current number of observations with suitable images.
Bild / Image: Basic algorithm that classifies species only based on image information without considering location.
Bild & Ort / Image & Location: Extended algorithm that also considers ecological site information for classification.
How to take good pictures
Image format
We use quadratic image formats in order to train the image classifier with a resolution of no less than 500×500 pixels. If incoming images do not have a quadratic format, quadratic center crops will automatically be taken for the analysis.
Image content
Images should be sharp and have a well-balanced light exposure. The plant species targeted should dominate and be located in the image center. Several photos showing different perspectives of an observation are desired, if possible with a focus on different organs, particularly inflorescence, leaves, and fruits, and taken from different distances, particularly covering groups of individuals, individuals, and organs. Moreover, observations from individuals of different lifestages are helpful, whereby the plant material should be alive/green. Blurry images or images with bad exposure, images of landscapes, and images of plant communities have to be filtered out and are therefore not desired.