Combining machine learning, ecological modelling and plant morphological characteristics to develop a tool for identifying plants of Switzerland
Philipp Brun
Patrice Descombes
Lucienne Claudine de Witte
2020 - 2022
Coopération FinancementCombiner l’intelligence artificielle, la modélisation écologique et les caractéristiques morphologiques des plantes pour développer un outil d'identification de la flore de Suisse
Objectif du projet
Les progrès récents en matière de classification d'images ont favorisé l'apparition d'applications mobiles conviviales aidant les amateurs à identifier des espèces à partir de leurs images, avec un grand potentiel pour la science citoyenne. Ces applications fonctionnent bien pour les espèces communes avec des caractéristiques morphologiques distinctes. Cependant, elles ont tendance à être moins performantes pour les espèces moins courantes et pour les groupes d'espèces morphologiquement très similaires. De nombreuses espèces de plantes suisses, par exemple, ne sont pas bien reconnues par les applications actuelles. Nous proposons ici une nouvelle approche combinant la reconnaissance d'images basée sur l'apprentissage automatique avec des méta-informations écologiques et morphologiques spatialement explicites pour identifier les quelque 4'000 espèces de plantes poussant en Suisse sur la base d'images géoréférencées. Cette combinaison devrait améliorer considérablement l'identification des espèces, car les nouvelles images ne sont pas seulement classées en fonction de leurs caractéristiques visuelles, mais aussi en fonction de leur plausibilité écologique et géographique. Ces développements seront d’une grande aide pour tous les botanistes suisses lors de l'acquisition et de la validation des données.

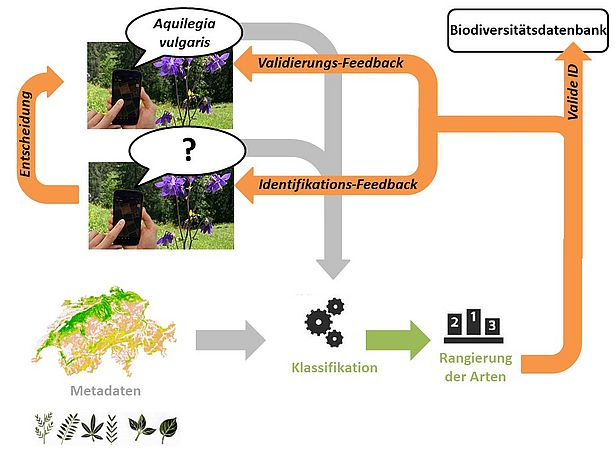
10 mai 2021
Ce week-end, nous sommes parvenus à finaliser la deuxième génération de nos réseaux neuronaux. Les nouveautés par rapport au mois dernier sont les suivantes:
- Inclusion de toutes les observations et images Info Flora prises du 9 mars au 23 avril.
- Inclusion des images de l'Atlas de la flore vaudoise comme images d'entraînement.
- Inclusion d'extraits d’images Info Flora effectués manuellement pour environ 1000 espèces.
Cet agrandissement de la base de données et l'amélioration de sa qualité ont permis d'accroître la précision des réseaux. Par exemple, le nouveau réseau de reconnaissance d'images identifie désormais correctement 74,4 % des images testées, et pour 91,1 % des images testées, l'espèce correcte figure parmi les cinq espèces que le réseau considère comme les plus probables. Lorsque l'image et les informations de localisation sont combinées, nous obtenons maintenant une précision de 78,3 % et une précision de 93,1 % dans les statistiques correspondantes. Lorsque le réseau de reconnaissance d'images dispose de deux images pour reconnaître l'espèce d'une observation test, l'espèce est correctement identifiée dans 85,3 % des cas. En outre, nous avons pu augmenter de 40 le nombre d'espèces pour lesquelles nous disposons de suffisamment d'images, atteignant ainsi 1699 espèces. Ces nouvelles espèces sont :
- Anemone blanda Schott & Kotschy
- Anthriscus caucalis M. Bieb.
- Armoracia rusticana G. Gaertn. & al.
- Asparagus officinalis L.
- Asplenium billotii F. W. Schultz
- Aubrieta deltoidea (L.) DC.
- Butomus umbellatus L.
- Camelina microcarpa DC.
- Cistus salviifolius L.
- Clypeola jonthlaspi L.
- Cotoneaster salicifolius Franch.'
- Diplotaxis muralis (L.) DC.
- Dorycnium herbaceum Vill.
- Draba tomentosa Clairv.
- Drosera ×obovata Mert. & W. D. J. Koch
- Euphorbia myrsinites L.
- Forsythia ×intermedia Zabel
- Galega officinalis L.
- Gentiana insubrica Kunz
- Hemerocallis fulva (L.) L.
- Himantoglossum robertianum (Loisel.) P. Delforge
- Hymenolobus pauciflorus (W. D. J. Koch) Schinz & Thell.
- Isopyrum thalictroides L.
- Lathyrus sphaericus Retz.
- Laurus nobilis L.
- Lonicera nitida E. H. Wilson
- Lythrum portula (L.) D. A. Webb
- Muscari armeniacum Baker
- Myosotis discolor Pers.
- Nigella damascena L.
- Peucedanum venetum (Spreng.) W. D. J. Koch
- Pisum sativum L.
- Potentilla heptaphylla L.
- Primula acaulis × veris
- Pteris cretica L.
- Quercus cerris L.
- Scilla siberica Haw.
- Sisymbrium irio L.
- Spirodela polyrhiza (L.) Schleid.
- Symphytum bulbosum K. F. Schimp.
- Thuja plicata D. Don
- Veronica praecox All.
- Viola collina Besser
(Trois espèces sont retombées en dessous de la limite de 30 observations avec images après modification de la base de données). Une vue d'ensemble actualisée de la précision des identifications au niveau de l’espèces peut être trouvée dans ce document. Vous trouverez de plus amples informations sur les statistiques et les espèces prioritaires à photographier dans l'onglet "Taxon prioritaires" de la section "Soutien au projet".
Il est important de noter qu'il s'agit de résultats préliminaires et qu'ils doivent être pris avec précaution. Au cours des prochains mois, nous continuerons à nettoyer et à ajuster minutieusement les données d'entraînement et surtout de test, de sorte que les valeurs de qualité, en particulier au niveau des espèces, peuvent encore changer de manière significative. Les informations permettant de savoir si le matériel d'image disponible est suffisant ou non sont déjà plus fiables.
Généralités
Pourquoi avons-nous besoin de l'aide des scientifiques citoyens ?
Afin de développer un algorithme fiable, nous avons besoin d'environ 100 images de haute qualité pour chaque taxon de plante suisse, qu'il soit indigène, naturalisé ou cultivé. À cette fin, nous utilisons les observations anonymisées de la base de données d’InfoFlora, qui contient actuellement environ 400'000 images de plantes. Pour quelques espèces très répandues, nous avons déjà beaucoup d'images, mais pour la grande majorité des espèces, le nombre d'images est bien inférieur à 100 (voir l'onglet "Taxons prioritaires"). Le succès de ce projet dépendra donc en grande partie du nombre d'images de plantes de haute qualité que nous recevrons des scientifiques citoyens tout au long de la saison 2021.
Comment vous pouvez nous soutenir ?
Ce projet est mené en collaboration avec InfoFlora qui fournit la base de données et l'infrastructure. Info Flora offre une plateforme conviviale permettant aux scientifiques citoyens de faire des observations d'espèces végétales sur le terrain, y compris des témoins photographiques, de manière standardisée. L’application smartphone FlorApp est la solution la plus simple et la plus utilisée pour récolter des images liées à des observations de terrain. Ceux qui préfèrent ne pas utiliser les appareils photo des smartphones pour prendre des photos de plantes peuvent compléter les observations faites sur le terrain de manière pratique à la maison, en utilisant le Carnet en ligne.
Comment la communauté bénéficie de votre aide?
Le classificateur que nous développons dans ce projet fera partie d'un module d'identification de FlorAppet sera donc librement accessible à tous. Ce module d'identification fournira une aide en temps réel à ceux qui s'intéressent à l'identification des espèces végétales sur le terrain et permettra aux botanistes citoyens d'améliorer efficacement leurs compétences. Plus nous recevrons d'images, meilleure seront les suggestions d’identification de l'algorithme.
Pâques 2021
Nous venons d'entraîner le premier ensemble de réseaux neuronaux et nous avons déjà atteint une précision de classification décente pour 1’659 espèces. En se basant uniquement sur les informations de l'image, le meilleur réseau identifie correctement 72,2 % des images de test, et pour 90,4 % des images de test, l'espèce correcte se trouve dans le « top 5 » (i.e. parmi les cinq espèces jugées les plus probables par le réseau). Si l'on inclut également les informations géographiques, les statistiques passent à 76 % pour les classifications correctes et à 92,4 % pour les correspondances parmi les cinq suggestions les plus probables. Même si ces chiffres sont encourageants, ils montrent également que pour de nombreuses espèces, les classifications ne fonctionnent pas encore bien. De plus, le matériel image est actuellement insuffisant pour toute évaluation dans le cas de près de 2’000 espèces. Un aperçu détaillé de la précision des classifications au niveau des espèces est fourni dans ce document. De plus amples informations sur les statistiques utilisées et les espèces prioritaires à photographier sont disponibles dans l'onglet "Taxon prioritaires" de la section "Soutien au projet".
Il est important de noter qu'il s'agit de résultats préliminaires qui sont appelés à être améliorés. Dans les mois à venir, nous allons nettoyer et adapter en profondeur les données utilisées pour entraîner les modèles informatiques et les données de test. Par conséquent, les scores de qualité peuvent changer distinctement, en particulier au niveau des espèces. L'information sur la disponibilité ou non d'images adéquates pour les différentes espèces devrait cependant restée inchangée.
Taxons prioritaires
Les espèces à photographier en priorité sont celles pour lesquelles nous disposons actuellement de moins de 30 observations avec des images appropriées ou qui ne peuvent actuellement être identifiées que de manière imprécise par le classificateur. Voici un fichier PDF listant toutes les espèces concernées, pour lesquels nous avons utilisé une précision Top1 du classificateur d'images seules de moins de 50% comme critère de qualité. Veuillez noter que les scores de qualité sont préliminaires et qu'ils seront probablement modifiés dans les versions ultérieures du classificateur. Dans les mois à venir, nous allons affiner les critères de priorité, en limitant les taxons prioritaires à quelques centaines d'espèes clés. Si vous préférez travailler avec les données de qualité sous forme de tableau afin d'être plus flexible dans la manipulation des données, vous pouvez utiliser ce fichier CSV de la liste de qualité complète.
Explications des documents
Afin d'évaluer la précision de la classification, nous sélectionnons au moins cinq images pour chaque espèce et les retirons du lot d’images d’entrainement, afin que l'algorithme ne les "voie" pas pendant la phase d'apprentissage. L'algorithme calcule ensuite, dans la phase de test, avec quelle probabilité il parvient à identifier correctement l’espèce illustrée sur les images retirées du lot. Nous en déduisons les statistiques suivantes (en gras : nom des champs du tableau) :
Top 1: L'espèce à laquelle l'algorithme attribue la plus forte probabilité est la bonne.
Top 5:L'espèce correcte se trouve parmi les cinq espèces pour lesquelles l'algorithme attribue les probabilités les plus élevées.
Ungenüngend Bildmaterial / Trop peu d’images (cercles rouges foncés) : Il y a moins de 30 observations avec des images appropriées disponibles pour l'espèce. L'adéquation des images est évaluée avant la classification dans une évaluation automatisée de l'adéquation. Les critères utilisés pour cette évaluation sont décrits dans l'onglet 'Comment prendre de bonnes photos'. Le diamètre des anneaux représente le nombre actuel d'observations avec des images appropriées.
Bild / Image : Algorithme de base qui classe les espèces uniquement sur la base des informations de l'image sans tenir compte de la localisation.
Bild & Ort / Image & Lieu: Algorithme étendu qui considère également les informations sur l’écologie du site pour la classification.
Comment prendre de bonnes photos
Format d'image
Pour entraîner le classificateur, nous utilisation des images de format quadratiques avec une résolution d'au moins 500×500 pixels. Si les images originales n'ont pas un format quadratique, le centre de la photo sera automatiquement extrait pour l'analyse.
Contenu des images
Les images doivent être nettes et avoir une exposition bien équilibrée. La plante cible doit dominer et être située au centre de l'image. Plusieurs photos montrant différentes perspectives d'une observation sont souhaitées, si possible en mettant l'accent sur différents organes, en particulier les inflorescences, les feuilles et les fruits, et prises à différentes distances, couvrant notamment des groupes d'individus, des individus et des organes. En outre, les observations d'individus à différents stades de leur phénologie sont utiles, le matériel végétal devant être vivant/vert. Les images floues ou mal exposées, les images de paysages et les images de communautés végétales doivent être filtrées et ne sont donc pas souhaitées.